Smart Text Chunking API
Contextual content retrieval made easy
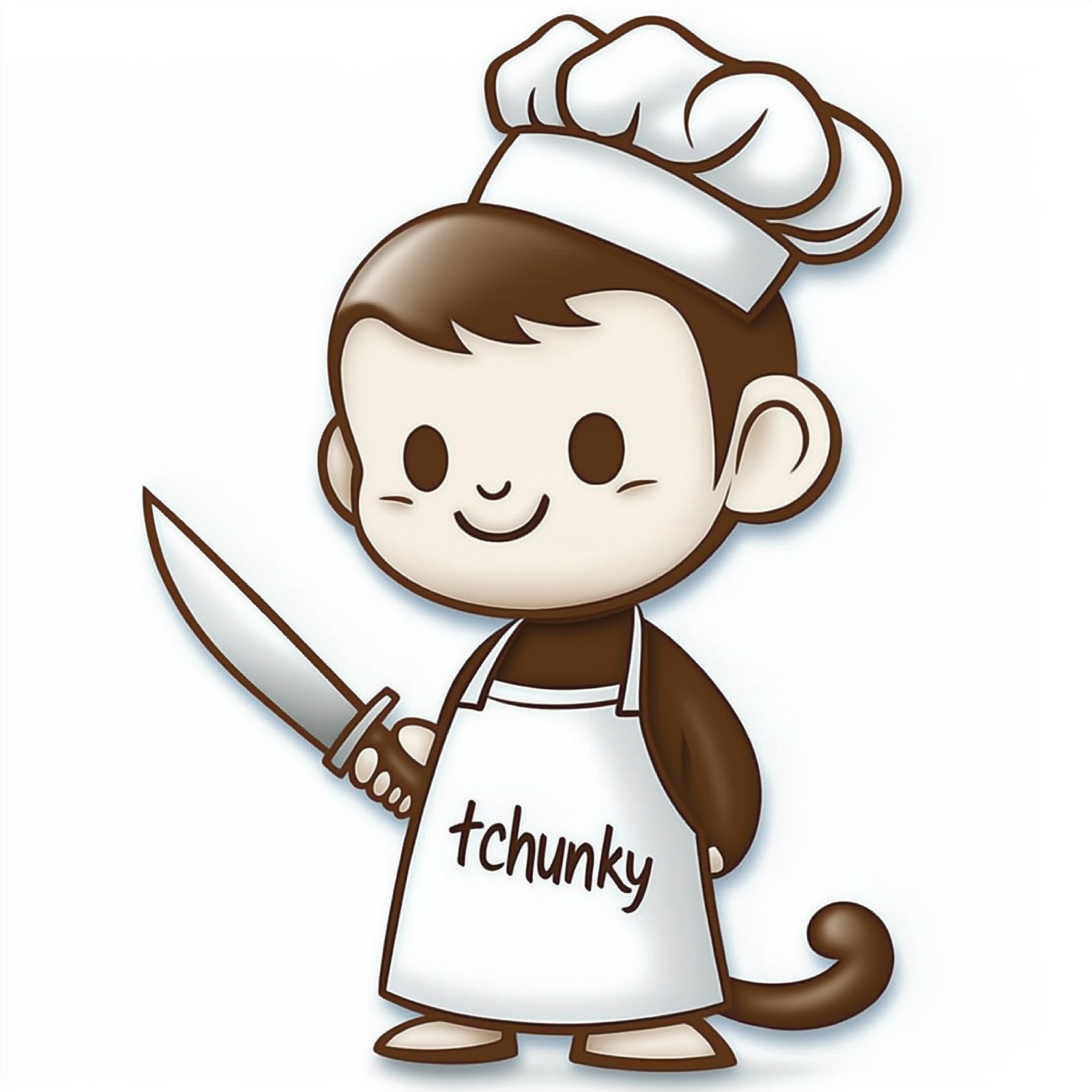
Mise en place
Long documents are cleanly sectioned into chapters or headings before chunking.
Slicing and Dicing
Splitting is based on semantic chunking, taking into account the meaning of the text.
Flavorful Options
Chunks optionally get context, keywords and timeframe attributes.
Books
Novels, technical manuals, textbooks, and non-fiction books often need to be broken down into chapters, sections, or paragraphs to retain the thematic coherence.
White Papers
Complex documents usually structured with introductions, problem statements, solutions, and conclusions, making it essential to break down by sections or key ideas.
Reports
Business or technical reports often have distinct sections like executive summaries, data analysis, recommendations, and appendices that should be embedded individually for clarity.
Websites, Blogs, and News Articles
Web content can be chunked by pages (landing, about, services), and on each page, sections like headers, body content, and footers are usually distinct in meaning.
Research Papers
Sections like abstract, literature review, methodology, results, and discussion provide natural breaks for chunking.
Legal Documents
Contracts, agreements, or policies, which often have articles, clauses, and sub-clauses that represent independent legal concepts.
Meeting Transcripts
These might be chunked by speaker or topic shifts, which allows for better retrieval of specific parts of the conversation.
Email Threads
Long email conversations can be divided by individual email or by specific topics within the thread for better semantic understanding.
Each of these document types has its own structure, and chunking them appropriately depends on understanding the natural breaks that make sense for embedding.